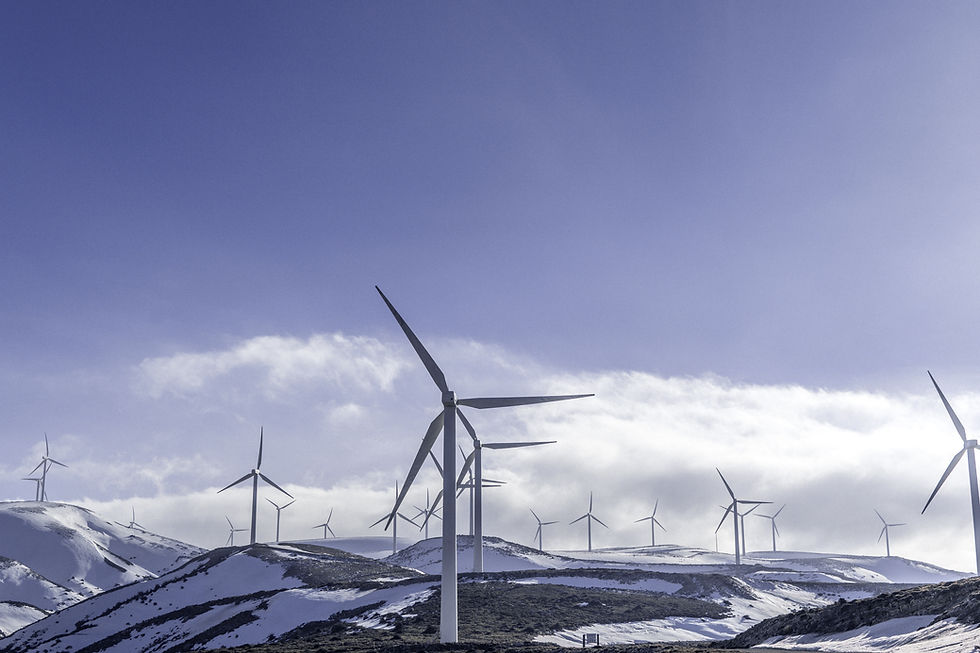
From Fossil Frustration to Amped Innovation: Sparking a Renewable Renaissance
Renewable energy is back in the spotlight, bolstered by a renewed surge in investor confidence and strategic global initiatives. By leveraging advanced mathematical modeling and intricate quantitative analysis, we can uncover and explore the evolving trends within the renewable energy market, providing a more profound understanding of its future potential.
I. Overview: Market Size and Growth Trajectory
The global renewable energy market is on a rapid expansion trajectory, projected to reach a staggering $1.5 trillion by 2025. This growth is driven by a Compound Annual Growth Rate (CAGR) of 6.1% from 2020 to 2025, as reported by Grand View Research in 2020. This CAGR is indicative of the increasing adoption of renewable energy technologies, spurred by a combination of escalating electricity demand, declining costs of renewable technologies, and supportive government policies worldwide.
Key contributors to this growth include:
Government Initiatives: Stringent government policies aiming to reduce carbon emissions, combined with substantial subsidies and incentives, have been instrumental in encouraging the shift toward renewable energy sources.
Rising Energy Demand: The global demand for electricity is growing exponentially, driven by urbanization, industrialization, and the increasing use of electronic devices and electric vehicles.
Technological Advancements: Innovations in energy storage, grid integration, and renewable energy technologies, such as wind, solar, and hydroelectric power, have significantly reduced the levelized cost of electricity (LCOE) from renewable sources, making them more competitive with traditional fossil fuels.
However, this optimistic outlook requires a more nuanced and detailed quantitative analysis to capture the market's complexities and forecast future trends with greater precision.
II. Advanced Quantitative Analysis
To gain a deeper understanding of the market dynamics, a time series analysis of the past five years’ renewable energy market data was conducted. This analysis aims to identify long-term trends, seasonal patterns, and short-term volatility that are critical for financial market participants and investors.
The ARIMA (AutoRegressive Integrated Moving Average) model was selected for this time series forecasting. ARIMA models are adept at handling non-stationary data, which is common in financial markets due to fluctuating market conditions and policy changes. The model is defined as follows:
X(t) = c + ε(t) + Σ [ α(i) * X(t-i) ] + Σ [ β(i) * ε(t-i) ]
where:
- p is the order of the AutoRegressive part
- d is the degree of first differencing involved
- q is the order of the Moving Average part
- ε(t) is white noise
After rigorous model fitting and evaluation using historical data of the energy index, the optimal ARIMA model was identified as (2, 1, 2), indicating that two autoregressive terms, one differencing operation, and two moving average terms were necessary for an accurate fit. The selection of this model was based on the minimization of the Akaike Information Criterion (AIC)Â and the Bayesian Information Criterion (BIC), both of which serve as measures of model quality. The model's performance showed that the residuals were approximately white noise, validating the model's adequacy in capturing the underlying data patterns.
Insights: The fitted ARIMA model revealed persistent patterns in the market, including cyclical fluctuations tied to policy changes, technological advancements, and investor sentiment shifts. This analysis indicates that, despite short-term volatility, the long-term trajectory for the renewable energy market is upward.
III. Monte Carlo Simulations
To further enrich the analysis, a Monte Carlo simulation was conducted using the residuals from the fitted ARIMA model. Monte Carlo simulations are invaluable for modeling the probability of various market outcomes, especially in the context of uncertainty and complexity inherent in the renewable energy market.
Simulation Details:
Assumptions: The residuals of the ARIMA model were assumed to follow a normal distribution. This assumption allows for the generation of random variables that mimic potential future fluctuations in the market.
Number of Simulations: 1,000 simulations were run to forecast the energy index over the next 12 months, generating a wide array of potential future paths for market performance.
Results: The simulated paths were analyzed to derive the 5th, 50th (median), and 95th percentiles, providing a range of probable outcomes.
Findings: Across all percentiles, the Monte Carlo simulation indicated a rising trend in the energy index. Even under conservative scenarios (5th percentile), the model suggests robust growth in the sector, reinforcing the notion that investor confidence in renewable energy is not only driven by climate change concerns but also by strong market fundamentals.
IV. Regression Analysis and Correlations
To identify the factors driving the growth of the renewable energy market, a multiple regression analysis was conducted, examining variables such as governmental investment in renewables (GI), electricity consumption (EC), carbon emissions (CE), and the price of non-renewable energy sources (PNE). The regression equation is formulated as follows:
The resulting regression equation:
RE = β0 + β1 * GI + β2 * EC + β3 * CE + β4 * PNE + ε
where:
- RE stands for Renewable Energy
- β0, β1, β2, β3, β4 are the coefficients
- ε is the error term
Key Findings:
Government Investment (GI): Statistically significant with a p-value < 0.05, indicating that increased government spending and subsidies in renewable energy projects directly correlate with market growth.
Electricity Consumption (EC): Also significant, reflecting the ongoing transition towards electrification in various sectors, including transportation and industry, which boosts demand for clean energy sources.
Non-Renewable Energy Prices (PNE): High prices for fossil fuels serve as a catalyst for the adoption of renewable energy, as they make alternative energy sources more economically viable.
Carbon Emissions (CE): While significant, the effect of carbon emissions on market growth is more nuanced. Although stricter emission regulations incentivize the adoption of renewables, this factor's impact is mediated by the global policy environment and technological advancements.
The model showed a high R-squared value, indicating that these variables collectively explain a substantial portion of the variation in the renewable energy market's performance.
V. Conclusion
Through detailed quantitative analysis using time series modeling, Monte Carlo simulations, and regression analysis, the outlook for the renewable energy sector appears bullish. This optimism is not solely based on environmental concerns but is firmly grounded in data-driven insights and economic factors, including government investment, rising electricity demand, and the competitiveness of renewable technologies relative to traditional energy sources.
Investors can thus base their decisions on robust statistical evidence that points to a growing market, albeit with the usual considerations for risk tolerance, market fluctuations, and investment horizons. The incorporation of advanced modeling techniques further validates the potential for continued upward momentum in the sector.
Investment Implication
Given the sector's upward trajectory, investment in renewable energy assets is well-supported by statistical analysis and market fundamentals. However, investors should remain vigilant, continuously monitoring policy changes, technological developments, and market trends to adapt their strategies accordingly. As always, this analysis is not financial advice but serves as an informed guide to the dynamics at play in the renewable energy market.
Note: The contents of this analysis are derived from mathematical modeling and statistical evaluations, serving to provide insights into market trends rather than direct investment guidance.